Monday, July 8, 2024
Generator Attribution in GenAI Images - “Laboratory” Benchmark and Accuracy Report
Mastering GenAI Attribution: A Comprehensive Benchmark for Image Generator Identification
Download This Dataset
Fill out this form with your information and someone from the Deep Media research term will grant you access: Click here!
Abstract
As AI-generated images flood our digital landscape, the ability to attribute these creations to specific generators has become crucial for maintaining digital integrity and trust. Deep Media AI, a trailblazer in Deepfake Detection, now turns its expertise to the challenge of GenAI Image Generator Attribution.
We are proud to introduce our GenAI Image Generator Attribution Lab Benchmark Dataset, a powerful new tool in the fight against digital misinformation and fraud.
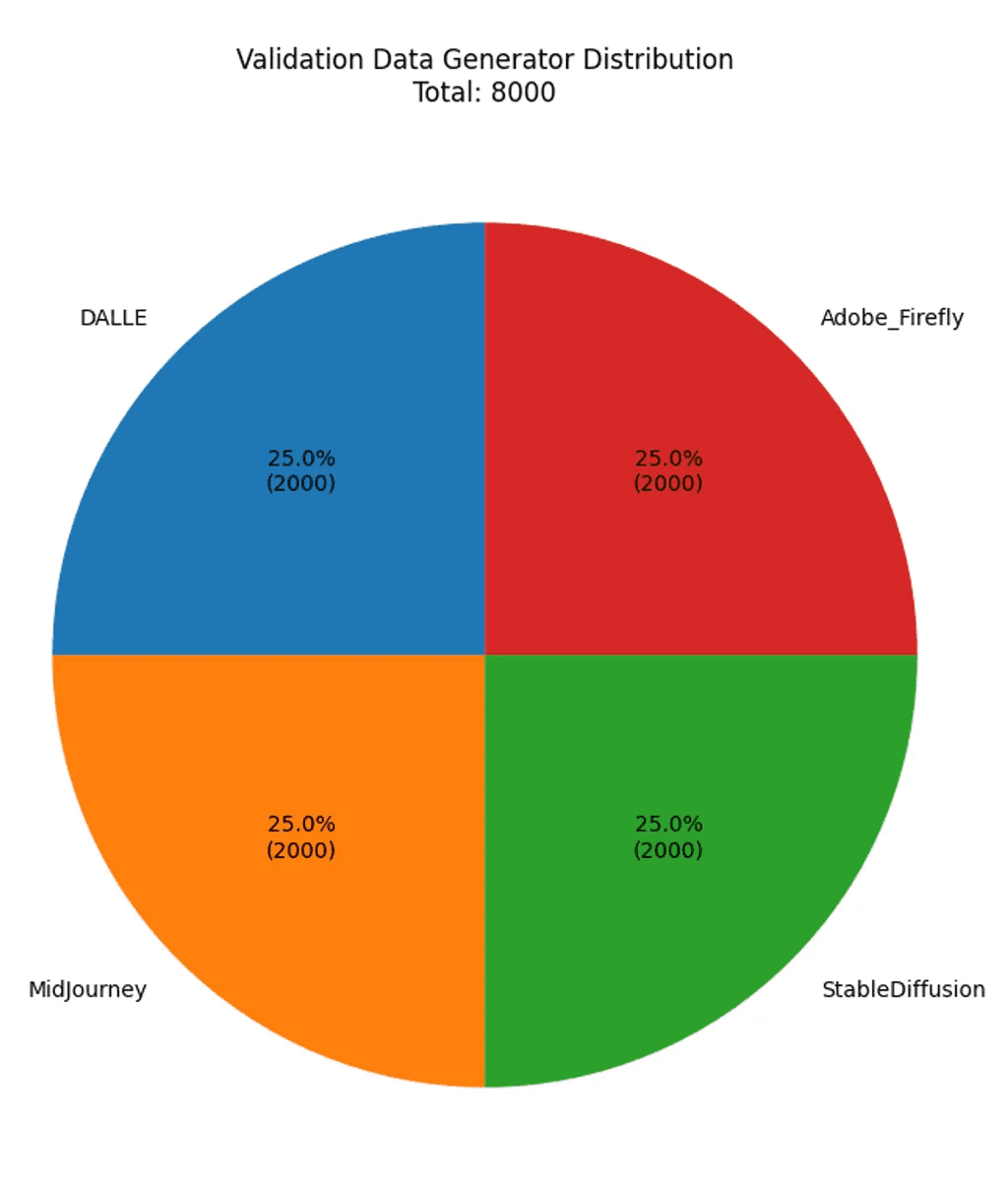
Key Features:
8,000 high-fidelity images, representing the most comprehensive and current collection of AI-generated content for attribution research
Equal distribution across four leading generators: DALL-E, Midjourney, Stable Diffusion, and Adobe, providing a balanced representation of current market leaders
Meticulously curated to represent a wide range of subjects, styles, and complexities, mirroring real-world use cases
Rigorous validation process, ensuring the integrity and accuracy of generator labels
Advanced metadata and embeddings provided for each image, facilitating cutting-edge machine learning approaches
This dataset is more than a research tool; it's a catalyst for innovation in the rapidly evolving field of AI-generated content attribution. As the line between human and AI-created content blurs, the ability to accurately identify the source of an image becomes paramount for industries ranging from journalism and law enforcement to digital rights management and social media moderation.
We call upon researchers, academic institutions, and industry leaders to harness this dataset in developing state-of-the-art attribution models. By fostering collaboration between academia and industry, we aim to create a safer, more transparent digital ecosystem where the provenance of visual content can be reliably determined.
Join us at the forefront of AI security and digital forensics. The future of online trust and accountability starts here.
On the Horizon: Expanding our benchmark to include emerging generators and modalities. As the generative AI landscape evolves, so does our commitment to staying ahead of the curve.
Results
Our image classification model demonstrates strong performance in distinguishing between images generated by different AI models (DALLE, MidJourney, StableDiffusion, and Adobe Firefly) and potentially real images. The overall accuracy of 90.54% indicates that the model correctly classifies more than 9 out of 10 images, which is a robust result in the complex domain of AI-generated image detection.

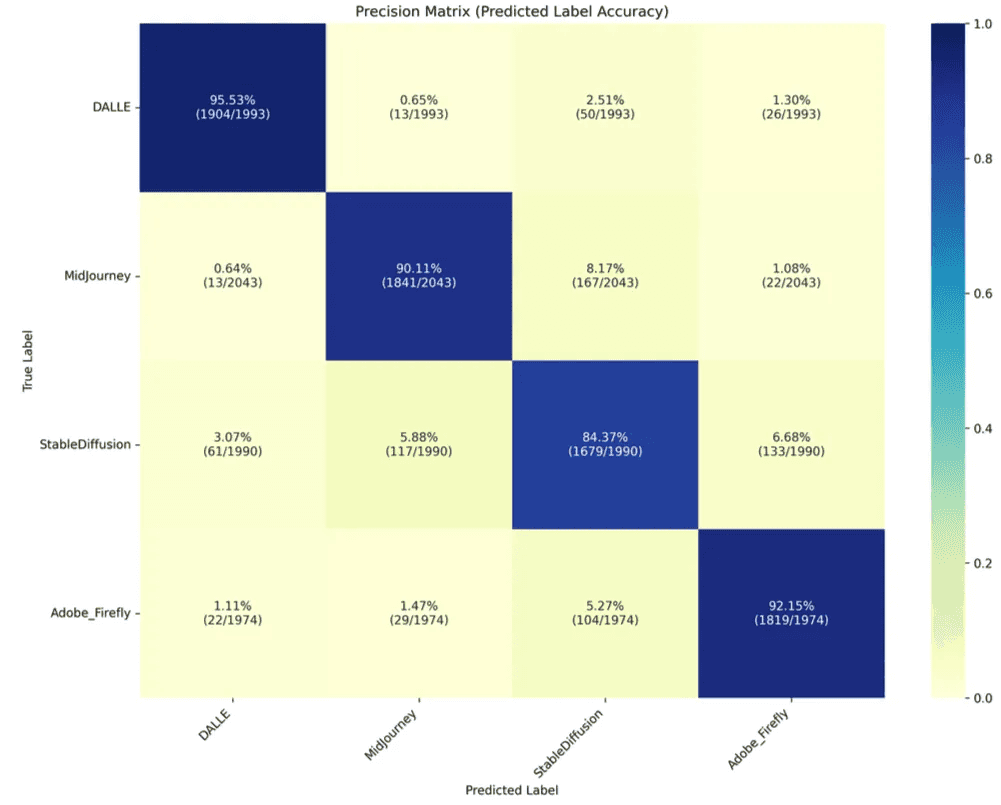
Examining the confusion matrix and classification report reveals several key insights:
Overall Performance: The model achieves high precision and recall across all classes, with a macro average F1-score of 0.91. This balanced performance suggests that the model is equally capable of identifying images from different generators without significant bias towards any particular class.
Generator-Specific Performance:
MidJourney: The model performs exceptionally well in identifying MidJourney images, with the highest precision (0.96) and recall (0.95) among all classes. This suggests that MidJourney-generated images have distinctive features that our model can consistently recognize.
DALLE: Close behind MidJourney, DALLE images are also well-identified with high precision (0.92) and recall (0.91). The model shows strong capability in distinguishing DALLE-generated content.
StableDiffusion: The model demonstrates good performance for StableDiffusion images, with a precision of 0.90 and recall of 0.92. While slightly lower than DALLE and MidJourney, these results are still impressive.
Adobe Firefly: The model's performance on Adobe Firefly images, while good (precision and recall both at 0.84), is noticeably lower compared to the other generators. This suggests that Adobe Firefly images may share more similarities with images from other generators or potentially with real images, making them more challenging to classify accurately.
Misclassifications: The confusion matrix shows that the most common misclassifications occur between StableDiffusion and Adobe Firefly, with 167 StableDiffusion images misclassified as Adobe Firefly and 133 Adobe Firefly images misclassified as StableDiffusion. This indicates a higher degree of similarity between the outputs of these two generators.
These results are particularly impressive given the challenging nature of distinguishing between different AI-generated images. The high accuracy and balanced performance across multiple generators demonstrate the model's robustness and potential for real-world applications in detecting and classifying AI-generated content.
Areas for Improvement:
Adobe Firefly Detection: Given the lower performance on Adobe Firefly images, future work should focus on identifying unique characteristics of these images to improve classification accuracy. This may involve fine-tuning the model with a larger dataset of Adobe Firefly images or exploring additional features that could help distinguish them from other generators.
Reducing StableDiffusion and Adobe Firefly Confusion: The model shows higher confusion between these two generators. Further analysis of the misclassified images could reveal common patterns or features that lead to this confusion, allowing for targeted improvements in the model's ability to distinguish between these two generators.
Expanding Generator Coverage: While the current model performs well on four major image generators, expanding the model to recognize images from additional AI generators would increase its utility and robustness in real-world scenarios.
Real vs. AI-Generated Distinction: Although not explicitly mentioned in the provided metrics, improving the model's ability to distinguish between real and AI-generated images across all generators remains a crucial area for ongoing research and development.
Adversarial Testing: To further validate the model's robustness, it would be beneficial to test it against adversarial examples or images intentionally designed to fool the classifier. This could uncover potential vulnerabilities and guide further improvements in the model's resilience.
In conclusion, our model demonstrates strong performance in classifying AI-generated images from different generators, with particularly impressive results for MidJourney and DALLE images. The areas identified for improvement, especially regarding Adobe Firefly detection and reducing confusion with StableDiffusion, provide clear directions for future enhancements to the model's capabilities.
Accelerating Research: Easy Access and Support
Fill out this form with your information and someone from the Deep Media research term will grant you access: Click here !
Ethical Innovation at the Forefront
We prioritize responsible AI development:
Strict usage guidelines prevent misuse
Rigorous anonymization protects privacy
Active monitoring ensures ethical compliance
By using our dataset, you're contributing to a safer digital future.
Let's Shape the Future of AI Security
Ready to push the boundaries of Deepfake detection? Contact research@deepmedia.ai today.
Together, we can build a digital world where synthetic media enhances creativity without compromising trust.
Your citations drive continued support and expansion of this critical resource.
Citation Information: When using the Deep Media validation set in research or publications, please cite it as follows: Deep Media. (2024). Deep Media Validation Set for Deepfake Image Attribution [Data set]. Deep Media. https://deepmedia.ai/ When citing the Deep Media validation set, please use the following BibTeX entry: @misc{deepmedia_validation_set_2024, author = {Deep Media}, title = {Deep Media Validation Set for Deepfake Image Attribution}, year = {2024}, publisher = {Deep Media}, howpublished = {\url{https://deepmedia.ai}} } We kindly request that you cite the dataset in any publications, presentations, or other works that make use of it. By properly citing the dataset, you help us track its usage and impact, which is essential for securing funding and resources to maintain and expand the dataset in the future. If you have any questions or require further assistance, please don't hesitate to contact our research team at research@deepmedia.ai. We look forward to collaborating with you and advancing the field of Deepfake image detection together.
Connect with Our Experts: Navigate the Path to Truth and Integrity.
